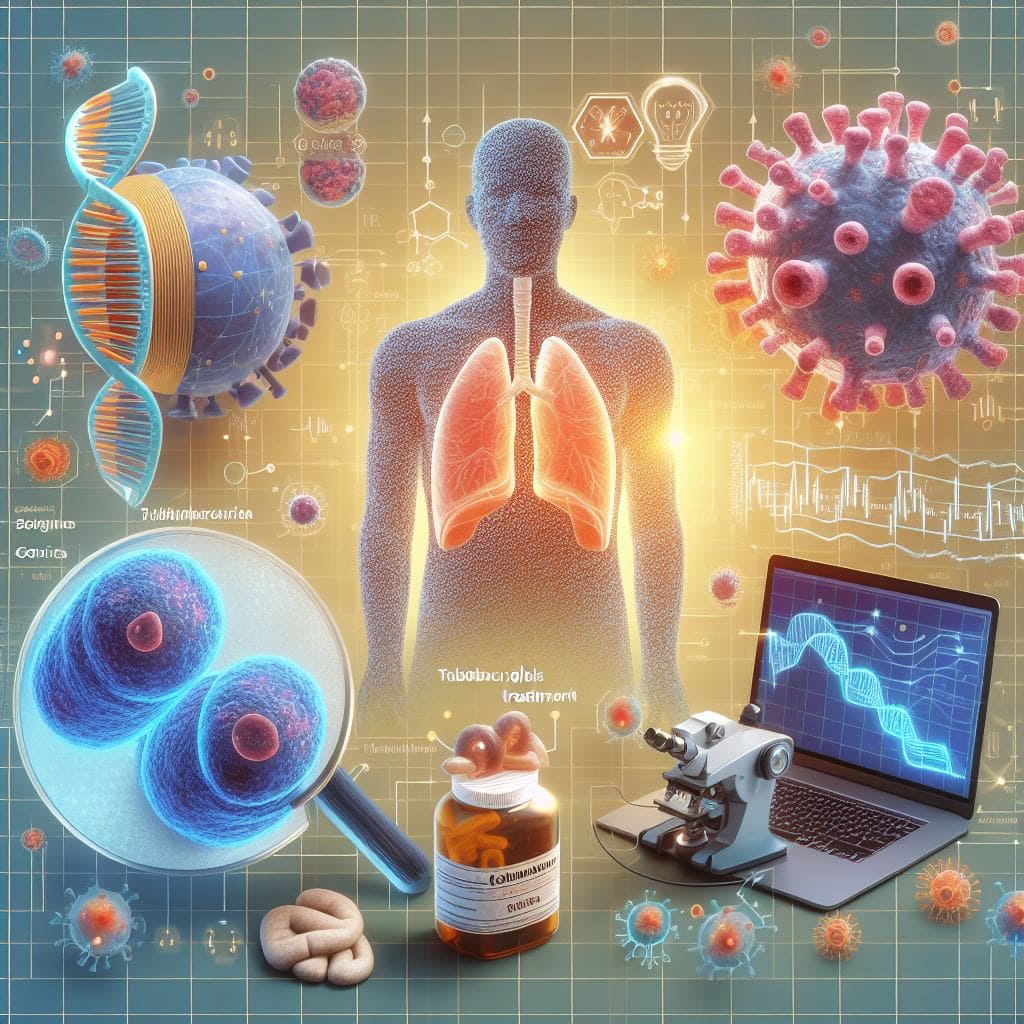
Multimodal AI model may guide personalized treatments for tuberculosis
AI approach helps researchers interpret large biomedical data sets.
AI approach helps researchers interpret large biomedical data sets.
AI approach helps researchers interpret large biomedical data sets to accurately predict tuberculosis treatment prognosis
A team of University of Michigan researchers, led by Sriram Chandrasekaran, Associate Professor, Biomedical Engineering, and postdoctoral fellow Awanti Sambarey developed a multimodal AI model to predict treatment outcomes of tuberculosis (TB) patients. Their analysis of real-world worldwide patient data may lead to personalization of TB treatment.
“TB is the world’s deadliest infectious disease, affecting millions of people each year. The goal of my lab is to develop new innovative solutions to stop the spread of drug resistant pathogens like those that cause TB,” Chandrasekaran said.
In the study, which was published in iScience on February 16, 2024, the researchers analyzed multimodal data including diverse biomedical data from clinical tests, genomics, medical imaging and drug prescription from TB patients. By analyzing data from patients with varying levels of drug resistance, they discovered biomedical features predictive of treatment failure. They also uncovered drug regimens effective against specific sets of drug-resistant TB patients.
“This was a highly interdisciplinary study involving U-M faculty in the School of Medicine, Public Health and Engineering,” Chandrasekaran said.
“Our multimodal AI model accurately predicted treatment prognosis and outperformed existing models that focus on a narrow set of clinical data,” Chandrasekaran added.
“A main finding was identifying drug regimens that were effective against certain types of drug-resistant TB across countries. This is very important due to the spread of drug-resistant TB,” added Sambarey.
Using AI, the team examined more than 5,000 patients. “This is real-world data we’re talking about, so patients from different countries have different admission protocols,” she said. “We worked with more than 200 biomedical features in our analysis; we examined demographic information such as age and gender as well as prior treatment history. We also noted if the patients had other comorbidities, such as HIV, and then we worked with several imaging features such as their X-ray, CT scans, data from the pathogens, drug-resistance data, as well as genomic features and what mutations the pathogen had.”
“It’s really difficult clinically to look at the data all together,” Sambarey said. “Typically, you would look at it separately. I think that’s where AI comes in handy. When clinicians look at all of this data, it can be overwhelming. Here, our research is able to identify the most meaningful clinical features.”
The team also studied the impact of the type of drug resistance that was present. “You can look at a specific snapshot of the data, such as genomic features and find what mutations the infecting pathogen had, and ask what some of the long-term treatment implications are,” she added.
Surprisingly, they found certain drug combinations worked better in patients with some types of resistance but not others, leading to treatment failure.
The researchers also found that drugs with antagonistic pharmaceutical interactions could result in worse outcomes. “Using AI to weed out antagonistic drugs early in the drug-discovery process can avoid treatment failure down the line,” Chandrasekaran noted.
“The AI model can also be eventually tailored to identify drug regimens suitable for individuals with certain comorbidities,” he added. “One thing I definitely want to say is instead of a one-size-fits-all treatment approach, we hope that the study of multimodal data will help physicians treat patients with more personalized treatments to provide the best outcomes,” said Sambarey.
This study was funded by various programs at the University of Michigan including the Precision Health Initiative, the Office of Vice-Provost for Research, the Michigan Institute of Clinical and Health Research (MICHR), the Michigan Medicine Pandemic Research Recovery program, and MCUBED. The authors are also grateful for the US National Institute of Health grants R56AI150826 and R01AI150826 for funding this study.
Co-authors include Professor Zhenhua Yang from the Department of Epidemiology and Professor Prachi Agrawal from the Department of Radiology. BME students Kirk Smith, Carolina Chung and Harkirat Singh Arora also contributed to this study.
Full citation: “Integrative analysis of multimodal patient data identifies personalized predictors of tuberculosis treatment prognosis,” Awanti Sambarey, Kirk Smith, Carolina Chung, Harkirat Singh Arora, Zhenhua Yang, Prachi Agarwal, and Sriram Chandrasekaran, iScience (2024). DOI: 10.1016/j.isci.2024.109025