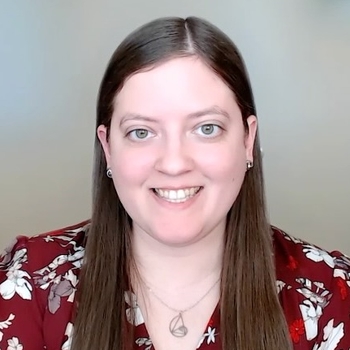
Anne Draelos, Collaborators Receive Research Scouts Funding
Research Scouts is an agile, low-burden funding program that gives money to scientists to invest in other scientists’ bold ideas.
Research Scouts is an agile, low-burden funding program that gives money to scientists to invest in other scientists’ bold ideas.
As neuroscientists seek to understand how neuronal populations respond to complex stimuli, it has become difficult to prioritize which stimulus parameters are most relevant for measuring in limited experimental time. For instance, even a small number of visual stimulus parameters (speed, direction, size) give rise to thousands of unique combinations: far too many to test exhaustively.
Anne Draelos, Assistant Professor, Biomedical Engineering and Computational Medicine & Bioinformatics and a member of the Michigan Neuroscience Institute, along with Elise Savier, Ph.D., Assistant Professor, Molecular and Integrative Physiology and Ophthalmology and Visual Sciences, and Christian Burgess, Ph.D., Research Assistant Professor, Michigan Neuroscience Institute and Assistant Professor of Molecular and Integrative Physiology, have received a Research Scouts funding grant.
Research Scouts is an agile, low-burden funding program from the Office of Research in the U-M Medical School which gives money to scientists (the “Scouts”) to invest in other scientists’ bold ideas. It’s an investment in the “Bold Science” objective of Michigan Medicine’s research strategic plan, “Great Minds, Greater Discoveries,” and is modeled on the Hypothesis Fund. The goals of the program are to:
·Spark new scientific conversations and connections
·Unleash the creativity of our scientists
·Test bold ideas that may otherwise go unexplored
·Have fun while facilitating new lines of investigation
From diverse disciplines across the Medical School, Research Scouts are given funding and are empowered and motivated to support their fellow researchers’ bold ideas. Scouts are searching for early-stage ideas that can transform our current understanding of a scientific concept or field, challenge common dogma, or are wildly new and imaginative.
The three collaborators propose to leverage machine learning and Bayesian optimization to estimate neural responses to such high-dimensional visual stimuli in real time, adaptively selecting each next stimulus for testing, which can yield exponentially faster results. Ultimately, these methods could bridge the gap between simplistic and complex stimulus spaces, giving scientists new insight into the underlying bases of how visual or other sensory stimuli are represented in the brains of behaving animals.
“The project itself is a collaboration, which is very exciting for me,” Dr. Draelos said. “I left my postdoc position with some preliminary data that show a particular kind of Bayesian optimization algorithm is effective at exploring the parameters underlying visual spaces. These are components that explain how we describe an image. For example, it has lines that move or it has dots with different sizes. We also have a method to analyze in real time the responses of neurons. Some neurons might respond a little bit, some neurons respond really strongly depending on what you show an animal. Because we can analyze the neural responses in real time, we can then use Bayesian optimization to turn around and say, okay, here’s the next stimulus you should display. Here’s the next thing you should look at. Here’s the next place you should search.”
Dr. Draelos added that these optimization studies have led to more efficient ways to approach this issue, especially with complicated parameter spaces. “You can’t show all possible kinds of images to a single animal,” she said. “To figure out what the neurons respond to would take too long. Instead, we have this more efficient approach that uses real-time neural responses and feeds it into this optimization routine to pick which next thing to show.”
In planning for longer-term studies, Dr. Draelos envisions expanding this research. “I think it would be really exciting to extend this beyond visual parameter spaces where we mostly understand the relationship between things like size and velocity to more complex parameter spaces like in olfaction, where different molecules are detected by different neurons. We could investigate how to design experiments that let us look at the relationship and parameter space between what an orange smells like versus what an apple smells like. All of this leads to a better understanding of the way circuits are flexibly encoding complicated things. The real world is complicated to navigate. A lot of our current understanding is necessarily limited by the amount of experimental time we have to test these hypotheses. These kinds of intelligent algorithms will help us figure out autonomously how to do many different tests without having to analyze the circuit manually.”
Dr. Draelos noted that the Research Scouts projects allow for the type of collaborative efforts that thrive at the University of Michigan. “It’s increasingly important to have strong collaborations among theorists, computationalists and experimentalists to enable these kinds of dream discoveries to happen,” she said. “In the interest of driving science and engineering forward, this is exactly the arrangement needed to be able to conduct these more transformational kinds of research.”