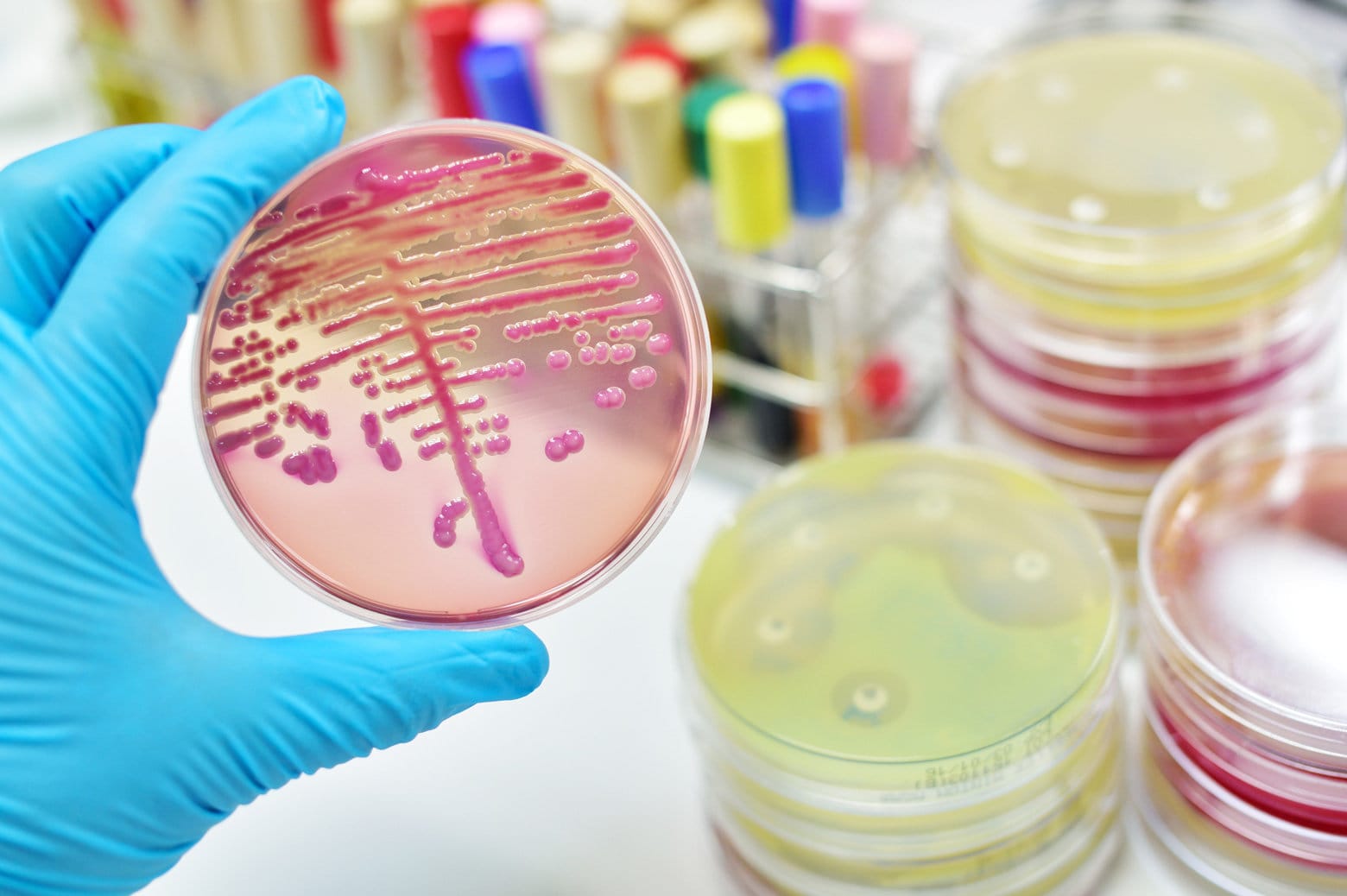
A ‘decathlon’ for antibiotics puts them through more realistic testing
Surprise findings could upend the current drug discovery approach for treating one of the most dangerous hospital-borne infections.
Surprise findings could upend the current drug discovery approach for treating one of the most dangerous hospital-borne infections.
The University of Michigan’s Sriram Chandrasekaran is using advanced computer simulations to study how different environments affect antibiotic performance. His most recent paper on this work, “Chemogenomic model identifies synergistic drug combinations robust to the pathogen microenvironment,” was published in December in PLoS Computational Biology. Michigan Engineering’s Jim Lynch recently sat down with Chandrasekaran, an assistant professor of biomedical engineering, for a Q&A.
Recent research has shown that a bacterium’s environment can have a big impact on the effectiveness of antibiotics. However, current drug discovery approaches have focused on the bacteria alone and not their environment. We may be losing some useful treatments from current drug discovery screens because we are testing in only one condition.
Harmful bacteria might be growing in the gut, lungs or on an implanted medical device. These all have different metabolic conditions. Even within the same location, individual bacterium in a colony, or “biofilm”, may encounter different levels of oxygen or other nutrients. Hence, antibiotics need to be effective in diverse environments.
Some environments significantly reduce the potency of antibiotics, thus giving bacteria more time to evolve resistance. To avoid the rise of resistant bacteria, we need to ensure the antibiotics being used can be effective in the environment the bacteria are in. Striving for that, however, greatly complicates the search for finding effective antibiotics, as there are a wide range of metabolic conditions that pathogens can encounter inside the body.
Instead of testing the efficacy of antibiotics in one condition, we are putting them through a decathlon, in a sense—testing them in a variety of environments. We studied the potency of antibiotics individually and in combinations under different growth conditions representative of conditions they may encounter inside the body. We utilized computer simulations to identify patterns we can use to forecast sensitivity. For example, some antibiotics that kill by breaking the bacterial cell wall might be effective in condition A, while those that block synthesis of DNA might be more effective in a different condition. We built a computer model using AI to find such patterns.
Since there are a large number of antibiotics and environmental conditions, it is impossible to experimentally screen all possible permutations. We used our computer model, which we call MAGENTA, to identify patterns in our experimental data and use it to forecast the impact of new conditions it had not seen before.
MAGENTA is the computational tool we developed for predicting the impact of environments on antibiotics. It’s an acronym for Metabolism And GENomics-based Tailoring of Antibiotic regimens. MAGENTA uses chemogenomics data, which provide information on the mechanism of action of antibiotics. Using chemogenomics, the MAGENTA tool can, for the first time, predict how a metabolic condition of interest changes the potency of antibiotics.
Up to 70 percent of the drug regimens we experimentally measured in E. coli showed significant difference in potency between different conditions. Notably, MAGENTA was able to accurately predict these changes in potency even in new conditions it had not seen before.
In addition to testing on E. coli, we used MAGENTA to predict the impact on different environments on antibiotic efficacy against the pathogen Acinetobacter baumannii—ranked as one of the most dangerous pathogens in hospitals worldwide by the Infectious Diseases Society of America. This pathogen is a frequent cause of drug resistant wound-, urinary tract- and pneumonia infections. A. baumannii infections display resistance to most antibiotics used in the clinic and new treatments are desperately needed.
We used MAGENTA to forecast the impact of nine diverse environments on 2,556 different drug regimens against this pathogen. MAGENTA identified 19 out of the 2,556 regimens to be synergistic across all these conditions. This represents just 0.74 percent of the total combinations screened. Thus less than one in 100 regimens are effective in all conditions against this pathogen. These 19 synergistic combinations uncovered by MAGENTA are novel and promising leads for treating A. baumannii.
There are certain combinations of antibiotics that are typically avoided in the clinic. For example: combinations involving antibiotics that merely slow down a bacterium’s growth with those that actively cause its death. These two classes of drugs are predicted to interfere with each other’s action (i.e. they are antagonistic), based on research performed in lab conditions. Surprisingly, we found that they were not antagonistic in all other conditions we tested.
Another surprise was only a very tiny fraction, less than 1 percent, of drug combinations were synergistic against the bacterium A. baumannii across all conditions. We can’t assume that drugs will work with equal efficacy in all conditions. This statistic underscores the need for methods like MAGENTA to match drugs with the right environment.
In the future, treatments will be influenced not just by pathogen type but also that pathogen’s environment. MAGENTA can help match drugs to the right environment.
We hope that MAGENTA can reduce the spread of drug resistance in two ways: by enhancing the effectiveness of existing antibiotics and helping re-discover antibiotics that are being ignored because they were ineffective in lab conditions.
Our approach still has some limitations that we plan to address in a future iteration. For example, in this study, we used MAGENTA to predict the impact of a fixed environmental condition. While this is different from the traditional lab condition, it is not exactly the same as the condition inside the body. The conditions bacteria encounter inside the body is highly dynamic (i.e. changes over time). We want to next simulate the impact of highly dynamic conditions on antibiotic potency.
Credits: